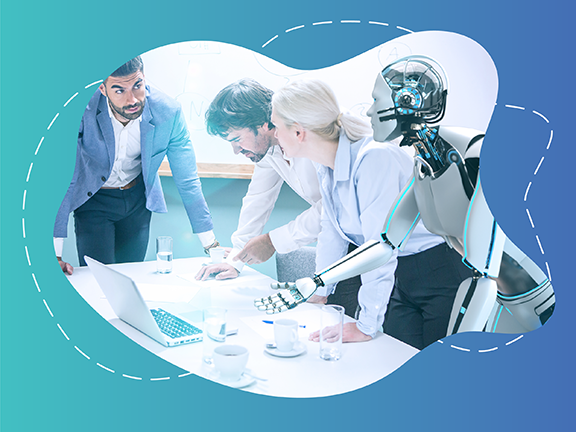
The Role of Machine Learning in Creating User-Centric Experiences
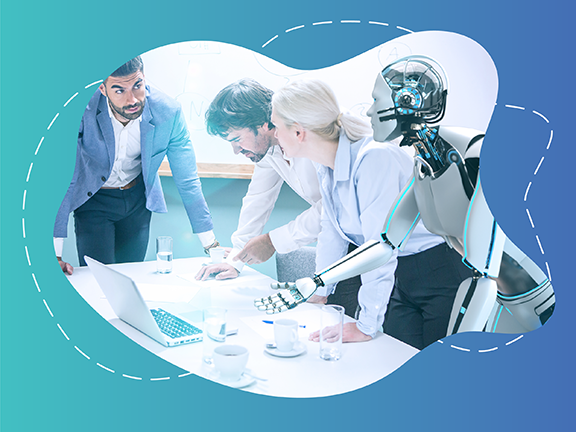
"Artificial intelligence will reach human levels by around 2029. Follow that out further to, say, 2045, we will have multiplied the intelligence, the human biological machine intelligence of our civilization a billion-fold." - Ray Kurzweil
This quote reminds us that AI is not something to fear, but rather a tool that can help us achieve incredible things, including designing experiences that delight and empower users. As ML continues to evolve and become more integrated into our daily lives, UX designers can stay in-touch with the latest developments and find ways to incorporate ML in their designs to enhance user experience.
To achieve your desired user experience, it's important to choose technology that aligns with it. Rather than immediately focusing on algorithms, consider how people currently complete the task. Determine what aspects are valuable and how you can improve the experience. During this process, you may discover a solution that doesn't require ML, which could be simpler to create and comprehend. This approach also applies to marketing: Emphasize the benefits for the user, rather than the technology behind the ML.
It is important that humans don’t completely expect ML to figure out what problems to solve. If you don't have a clear understanding of a human need, even the most powerful system you build may only address a minor or non-existent issue. Therefore, it's still necessary to conduct research and analysis such as contextual inquiries, interviews, surveys, and analyzing customer support tickets and logs to identify and solve problems or unmet needs. Machine learning alone cannot determine what issues require attention. As UX Designers, we already possess the necessary tools to aid teams, regardless of the technology used.
With the main driving force being human insight, here are a few ways machine learning can be used to improve user experience:
- Contextual understanding: ML algorithms can be trained vastly to understand the context in which a user is interacting with a product or service. Whether the experience is positive or negative, ML can be used to identify patterns. These patterns can then be used to design more personalized and adaptive experiences that are tailored to the specific needs/wants of a user.
- Emotional recognition: ML can be used to recognize and respond to users’ various set of emotions. This allows more empathetic and human-like interaction between the machine and the user. When a machine detects that a user is feeling confused, frustrated, or angry it can offer support or resources for the user.
- Continuous learning: ML can be used to enable products and services to continuously learn and adapt based on the user’s changing behaviors/needs/goals. This would ultimately allow for more efficient experiences that align with evolving trends based around the user thus resulting in an overall more positive user experience. Continuous learning is one of the most promising areas of ML within the field of UX.
- Predictive design: ML can be used to predict how users will interact with a product or service, which would allow designers to optimize and personalize the user experience before the product itself is deployed. Although actual analysis with real users is recommended, this can give designers a glimpse of potential trends they may expect to see when a product is undergoing user testing. With the combination of both ML and real user testing, more successful products and services can be produced.
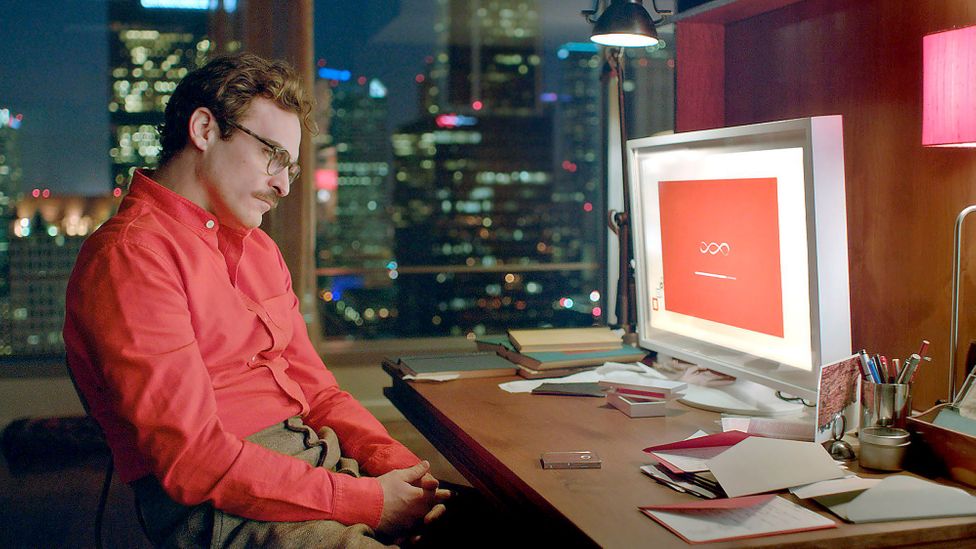
Although machine learning is great, we must understand the limitations of the technology. Machine learning models are not always accurate and can make mistakes, especially when they are trained on biased or potential data that is incomplete. Biases can occur when the data used to train the algorithm is not representative of the entire population or when it's collected in a way that skews the results. Incomplete data can lead to a lack of context or insufficient information of the entire population, which can lead to incorrect conclusions. It's crucial to recognize these limitations and to carefully consider the potential impact of machine learning on the user experience. For example, if a machine learning model is used to predict user behavior, it's important to recognize that these predictions are not always accurate. An inaccurately predicted behavior could lead to frustration and disappointment for the user. Similarly, if machine learning is used to personalize content, it's important to recognize that over-personalization can be invasive and even creepy, leading to a not so positive user experience. Boundaries must be created.
To avoid the potential issues associated, it's important to carefully consider the data used to train the machine learning model, and to constantly monitor and update the model as new data becomes available. This would help keep up with evolving trends. It's also essential to include human oversight and intervention to correct mistakes or biases that may arise. By understanding the limitations of machine learning and carefully considering its potential impact on the user experience, UX designers can ensure that they are creating products and services that truly benefit their users.
In conclusion, as ML continues to evolve, a brilliant and unique opportunity is created for UX designers to enhance their craft. However, it is crucial to remember that ML is a tool and not a solution to all our problems. The human insight is the primary driving force behind all things related to effective UX design.